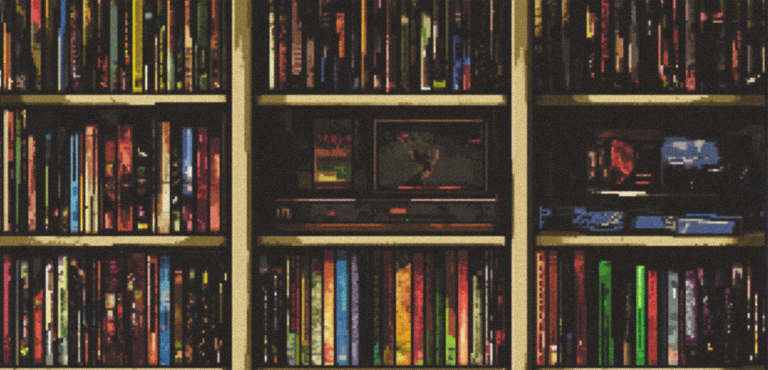
The Beginners Guide to AI Terminology
We won’t waste much time explaining the transformative qualities of AI, nor just how many orgs are using buzzwords across product suites and C-suite presentations. AI is in the spotlight, but the technology’s depth lies in a complex web of specialized terminologies and concepts. To fully understand AI’s potential and implications, this guide serves as your entry point to the world of AI terminology.
Artificial intelligence (AI): The term Artificial intelligence, or AI, was introduced by John McCarthy in the 1950s. It is focused on creating machines that can think and solve problems like humans. Today, the term broadly refers to any software capable of recognizing patterns within data.
Artificial General Intelligence (AGI): Artificial General Intelligence (AGI) refers to an AI capable of understanding, learning, and utilizing knowledge in various tasks and domains. It can be used in many situations, showcasing cognitive flexibility, adaptability, and general problem-solving capabilities. The key distinction is that standard AI operates based on pre-determined rules and algorithms. On the other hand, AGI has the capacity to learn, reason, and adapt without pre-defined rules, much like humans.
AI Hallucinations: AI hallucinations refer to instances where an AI model produces incorrect, deceptive, or nonsensical content yet represents it as factual. For example, a language model might claim that a fictional character like Sherlock Holmes was a real detective.
Bias: Machine learning bias, or AI bias, occurs when algorithms consistently generate prejudiced results. This bias can stem from an AI system’s code or the data it is trained on. And it has the potential to impact decision-making. For example, facial recognition systems might struggle to identify people with darker skin, and loan approval systems could favour certain demographics over others.
Black Box AI: Black box AI refers to any AI system where the inputs and operations are not accessible or understandable to the user or other relevant parties. A black box refers to a system that we cannot see inside or understand.
Cognitive Computing: Cognitive computing is a form of AI that mimics human thought processes and problem-solving abilities. The goal is to create computer systems that can understand, learn, and interact with people more naturally and intuitively. These systems can analyze large datasets, identify patterns, and make context-based decisions.
Machine learning: Machine learning is a subset of AI that enables software programs to improve their prediction accuracy automatically without direct human intervention. For instance, Amazon uses machine learning to suggest products to customers based on their past purchases and browsing history.
Model: An AI model is a machine learning algorithm trained on data and deployed for use.
Multimodal AI: Multimodal AI is a form of AI that can understand and work with multiple forms of information, such as text, images, audio, and other modalities. This is a powerful capability because it allows the AI to understand and communicate in diverse ways, providing a more holistic and nuanced understanding of tasks and information. For example, a multimodal AI translator can change Japanese comics into English by understanding both the words and the pictures.
Neural Network: A neural network is a computational model with a layered structure of the human brain’s neurons. It consists of interconnected processing elements called “neurons” that work collaboratively to generate an output. Neural networks typically have an input layer, an output layer, and, in many cases, one or more hidden layers that transform the inputs into a representation the output layer can use.
Neural networks are used for many things, like image recognition, predictive modelling and natural language processing. Since 2000, they have been instrumental in reading the handwriting on checks, changing spoken words to text, predicting the weather and recognizing people’s faces.
Prompt Engineering: Prompt engineering involves crafting input for AI systems that yield the best possible outputs. A prompt engineer crafts these inputs for large language models (LLMs), ensuring chatbots accurately understand and respond to user requests. They also play a vital role in identifying and addressing potential risks within the model.
Training Data: Training data consists of information utilized to train a machine learning model. It is like providing examples for a child to learn from. AI models need lots of training data to recognize patterns and develop their abilities.
Token: Tokens are essentially text segments, ranging from whole words to parts of words or even single characters. These pieces of text are broken down into tokens for analysis in the context of LLMs. This allows the model to understand the relationship between tokens and formulate responses accordingly. The ability of a model to handle a larger number of tokens simultaneously can enhance the quality of its output.
Distilled
As AI advances, mastering this specialized vocabulary will be crucial for keeping pace with the technology’s profound impact. This guide has equipped you with the building blocks to navigate the buzzing world of AI. So, dive deeper, ask informed questions, and participate meaningfully in discussions around the future of this transformative field. With this knowledge, you can now explore the intricate applications of AI with greater clarity and confidence.