Article
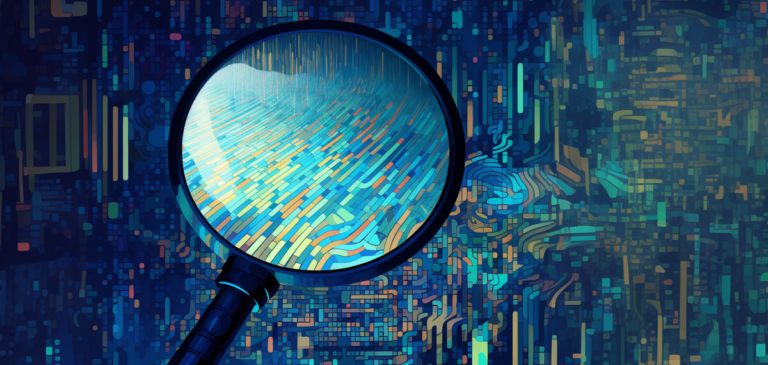
The Essential Data Quality Plan for IT Managers
Though the title may suggest otherwise, data security and quality are not a game to try out new strategies and hope one succeeds. Every IT Manager now has the vital task of safeguarding the quality and integrity of their org’s data assets – and this data is at the crux of intelligent decision-making, strategic planning, and game-changing innovation.
Data quality is all about the condition of your qualitative or quantitative data; top-quality data has no errors or inconsistencies and is just reliable and complete information. It can streamline decision-making, boost operational efficiency, increase customer satisfaction, and spark innovation.
How do you keep the data quality ship abreast without putting a spanner in the works of information flow or innovation? Let’s dive into this in-depth data quality plan we put together on mastering data management amidst the current digital climate.
Fundamentals of creating your unique data quality plan
According to IBM, and several other leading tech organisations, data quality is typically based on the following six pillars:
- Accuracy: The data should be spot on and free from errors.
- Consistency: The same piece of data should match across different systems.
- Completeness: All necessary data should be recorded and on hand.
- Timeliness: Data must stay up-to-date so it can be used when making decisions.
- Relevance: Data complexity aligns with its purpose so it can be easily leveraged.
- Uniqueness: The data should be dependable and without duplicates.
Operational strategies for creating your data quality plan
First up, you need to create a data governance framework. This framework should lay down the law for handling data initiatives, including standards for data entry and updates. Regular data auditing checks for data errors or inconsistencies can help you nip problems in the bud before they escalate. Reviewing data in an auditing process also reinforces an org’s adherence to regulations.
The use of data quality tools also significantly simplifies your plan. These software tools can automate data cleansing and validation processes, eliminating human error and cranking up efficiency. And last but not least, training your staff is vital. All employees should understand why data quality matters and the role they play in maintaining it. They specifically should know that data data issues must always be reported and to never stray from established data processes.
Examples of good (and bad) data quality practices
The good: Several companies have scored big by prioritising data quality, while others have suffered big losses due to poor-quality data. For instance, American Express utilized high-quality data to glean insights into customer behaviors and preferences. This strategy has enabled American Express to customize its services in a way that enhances customer satisfaction and loyalty.
The bad: Maintaining top-notch data quality has its challenges. You could face anything from data entry errors and inconsistent data formats to outdated information and system compatibility issues. Each problem has its unique headaches and needs a bespoke approach. And let’s not forget the sheer volume of data generated by modern businesses. With massive amounts of data flooding into your systems every second, maintaining data quality becomes a proper uphill battle. But despite these hurdles, keeping high data quality is crucial for business success.
The ugly: Samsung’s data entry error that cost the company $300 million. Despite experiencing a costly setback due to a lack of protocol, the tech giant learned a valuable lesson. Bear in mind that failing to comply with data laws such as the UK’s Data Protection Act or the EU’s General Data Protection Regulation (GDPR) can land you in hot water. The consequences of non-compliance include hefty fines, reputational damage to your organisation, and loss of customer confidence.
The Future of data quality management
Data quality management is moving toward an increased reliance on advanced technologies like AI and machine learning. More machine tools are being leveraged for automation in data cleaning and validation processes, which potentially enhance efficiency and accuracy in data management in the years to come.
Furthermore, as we progress further into the era of Internet of Things (IoT), the importance of data quality is becoming even more crucial. As countless devices connect to the internet and generate vast amounts of data, ensuring this data’s quality is increasingly important.
Distilled
Remember, creating a data quality plan isn’t a singular task – it’s an ongoing process that requires continuous monitoring, regular updates to strategies and technologies, and constant vigilance. By staying on the front foot with your approach to data quality management, you can ensure the integrity of your organisation’s data assets and keep the trust of your customers and stakeholders.